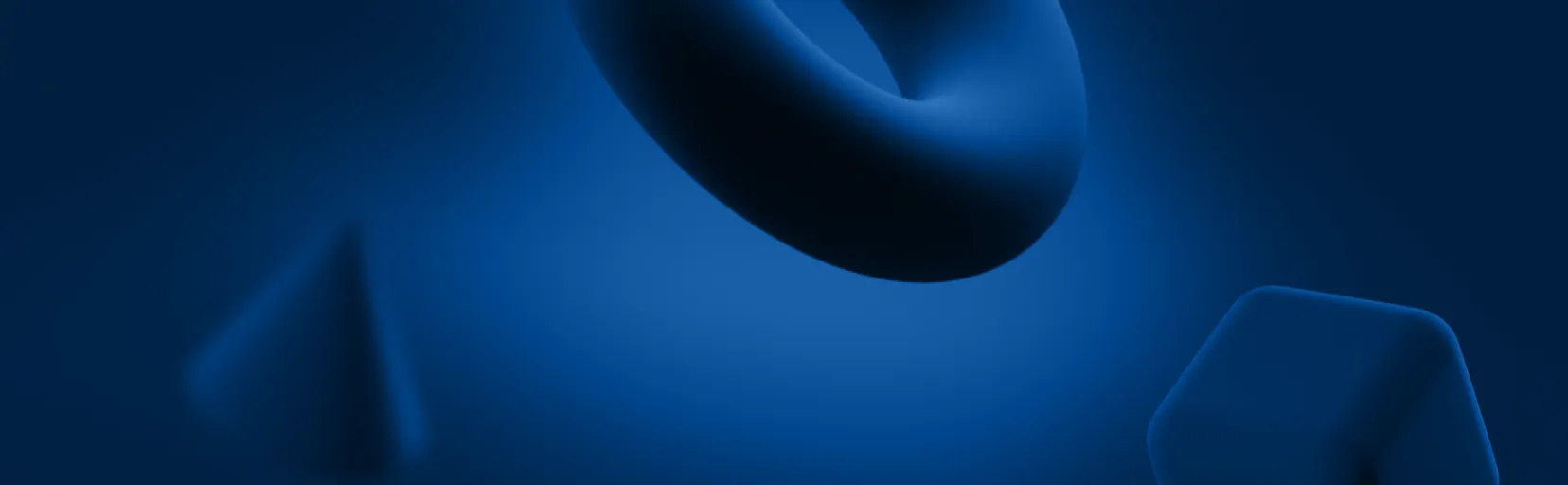
Efficient environment perception and reconstruction: Simplifying and compressing point clouds without the loss of meaningful information
Project data
Department in charge
In today's autonomous world, the transmission and interpretation of large amounts of information describing the environment is a key issue.
The research aims to make self-driving cars, robots, and drones work more efficiently. This requires more efficient processing and storage of the point cloud data (3D points recorded by LiDAR and other depth sensors) needed to sense the environment accurately. The problem is that this data can be very large, making it difficult to store, transmit, and process. The goal is to develop more efficient compression techniques that preserve the necessary information in point clouds while reducing storage requirements and processing overhead.
More efficient point cloud compression and reduced processing computational requirements could facilitate the wider adoption of self-driving vehicles, robots, and drones in everyday life, with several benefits, such as safer transport, more efficient logistics and transportation, or rescue and search and rescue operations.
The research is, therefore, the key to the wider deployment of autonomous systems. Developing effective compression techniques is essential to make these technologies available to everyday people. In addition, research contributes to national and international scientific and technological progress.