How to allocate the prize money in Formula One?
One of the most important tasks of governing bodies in sports is to distribute the revenue generated by all participants in a fair way. László Csató, a research fellow of the Laboratory on Engineering and Management Intelligence, Research Group of Operations Research and Decision Systems – together with his PhD student, Dóra Gréta Petróczy (Corvinus University of Budapest) – has written a paper on this topic entitled “Revenue allocation in Formula One: a pairwise comparison approach”, which has recently appeared in International Journal of General Systems.
Professional sports leagues and championships generate billions of euros. However, its allocation among the contestants is often burdened with serious legal disputes centred around unequal shares and the possible violation of competition laws. Consequently, constructing allocation rules which depend only on a few arbitrary variables, and are relatively simple, robust, and understandable for all participants, poses an important problem of academic research.
Formula One (Formula 1, or simply F1) is the highest class of single-seater car racing. A Formula One season consists of several races taking place around the world. The drivers and constructors accumulate points on each race to obtain the annual World Championships, one for drivers, and one for constructors. Since running a team costs at least 100 million US dollars per year, the distribution of Formula One prize money (1,004 million US dollars in 2019) can substantially affect competitive balance and the uncertainty around the expected outcome of races.
Since the money allocation is based on the ranking of the constructors, it is important to apply a robust and reliable ranking procedure– but the current points scoring system fails to satisfy this criterion. The long list of historical Formula One World Championship points scoring systems highlights the arbitrariness of this method and suggests that the relative importance of the different positions in a race remains unclear.
Inspired by these discrepancies, the authors have aimed to outline a formal model to share Formula One prize money among the teams in a meaningful way. Their proposal is based on pairwise comparisons and has strong links to the Analytic Hierarchy Process (AHP), a famous decision-making framework. In particular, they construct a multiplicative pairwise comparison matrix from the race results. Contrary to the Condorcet-like methods, it is not only said that a team is preferred to another if it has better results in the majority of the races, but the intensity of these pairwise preferences are also taken into account. Two popular weighting methods, the eigenvector method, and the row geometric mean, are considered to compute the revenue share of each team. The values of the pairwise comparison matrix depend on a parameter, which is shown to control the inequality of the allocation. Since the ranking of the teams by the eigenvector method is not independent of this parameter, the row geometric mean procedure has more favourable axiomatic properties in this setting. Historical data can uncover how the parameter influences the expected inequality of the revenue allocation, thus the decision-maker is able to fix the rules of the distribution before the competition is conducted.
The proposed technique has an important advantage over the official points scoring system of Formula One as it is independent of the somewhat arbitrary valuation given to the race prizes. Besides offering a novel way for sharing Formula One prize money among the constructors, the methodology can be applied in any area where resources/revenues should be allocated among groups whose members are ranked several times. Potential examples include further racing competitions, combined events in athletics (heptathlon, decathlon), the performance of countries in the Olympic Games, schools on the basis of student tests in various subject areas, or even workplaces where individual contributions on various projects are ranked.
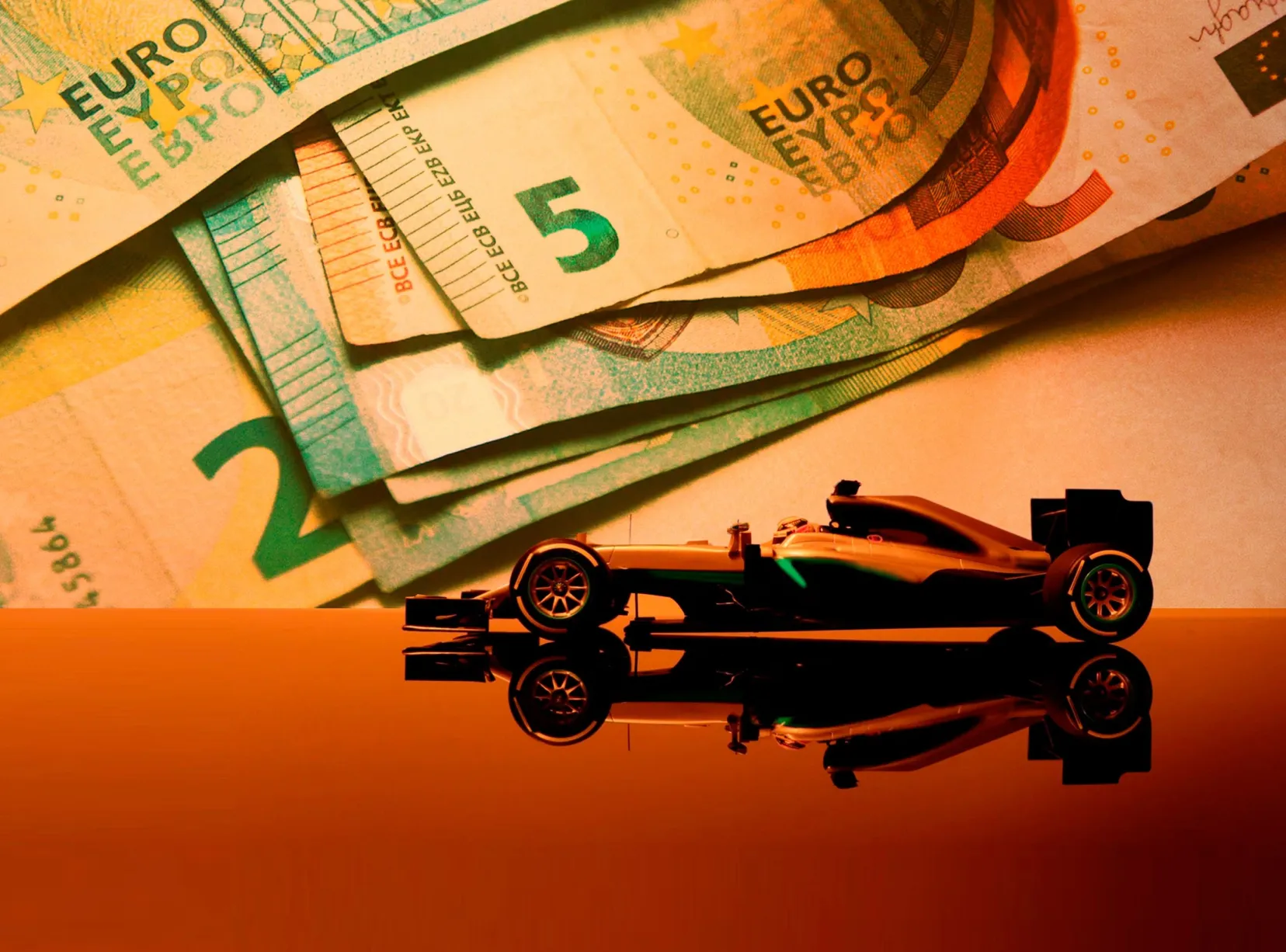